Does Your Company Have These Data Issues?
Why You Need a Data Governance Framework
The amount of data in the world is increasing exponentially, and timely access to data has become a prerequisite for making business decisions. Managing, cleaning, processing, and analyzing this data is incredibly complex for companies of all sizes. Making decisions based on faulty data can lead to huge downside with issues across practically all areas of a business. Anyone who has worked for an organization with unreliable data can attest to the frustration and the strategic and day to day blind spots this facilitates. With this in mind, data governance is an important guardrail on data collection and analytics.
What is Data Governance?
Data governance is a set of processes, policies, and guidelines that ensure high data quality, data management, and data security within an organization. It encompasses the practices and principles related to data management, data privacy, data security, and data utilization.
Data governance helps maintain accurate, consistent, and reliable data by defining data standards, data validation rules, and data quality metrics. This ensures that the data used in decision-making is trustworthy. It establishes data security measures and compliance with data protection regulations (such as GDPR, HIPAA, or CCPA). Data governance defines who can access, modify, and delete data and sets up protocols for data protection.
Data governance also helps organizations better manage the vast amounts of data they collect and store. It defines how data is collected, stored, organized, and maintained throughout its lifecycle. This involves data classification, data archiving, data retention policies, and data lineage.
All major data assets need an owner to oversee them within the organization; data governance assigns ownership and responsibility for data. It clarifies who is accountable for data-related decisions and ensures that data-related decisions align with business objectives. Similarly, there may be data stewards assigned. Additionally, data governance helps define how data can be used and shared within and outside the organization. This includes guidelines for data sharing, data sharing agreements, and data access controls.
In larger organizations, discovery can be a challenge. It’s often difficult to know where to find the right data when there are multiple business units and different teams involved. Data governance can help by creating metadata standards and catalogs to help users discover and understand the data available in the organization. This facilitates data discovery and utilization, and provides a set of clear universal criteria for those searching for information.
The Importance of Data Governance
Data governance is important even for smaller organizations because it helps ensure security and privacy, mitigates risks associated with data breaches and non-compliance, and ensures that companies are making decisions based on accurate data.
Without data governance, data can become siloed within different departments or teams, making it challenging to access and share data across the organization. This can result in duplicated efforts and a lack of a unified view of customer and business data. In other situations, different teams can use different data sources and arrive at different viewpoints and even actions around the same topic, due to data issues. Data governance helps keep different stakeholders on the same page.
Integrations can also quickly become an issue for companies using multiple different software tools and systems for overlapping functions. Without proper data integration, these systems can’t communicate effectively, leading to fragmented data. Data redundancy, where the same data is stored in multiple places, can lead to confusion and wasted storage resources. It can also make it difficult to determine which data is the most up-to-date and accurate. In many cases, these different systems can often produce different numbers for the same items, forcing impacted teams to reconcile data and trace errors. (when it comes to sales compensation data, Palette automates this process by reconciling data from your CRM and billing system, and proactively identifying and tracing errors in commissions).
Data is crucial in business decision making because it provides the foundation for informed, data-driven choices. It enables businesses to gain insights into customer behavior, market trends, and internal operations. With data, organizations can measure performance, identify opportunities, and detect potential issues. By leveraging data analytics and reporting, businesses can make decisions that are more accurate, efficient, and aligned with their strategic goals. Poor data quality can result from incomplete, inaccurate, or outdated information. This can hinder decision-making, lead to customer dissatisfaction, and undermine trust in the data. In addition to inaccurate data, the absence of a comprehensive data governance framework can result in data chaos. Data chaos is a situation where there are no clear guidelines for data access, usage, or ownership. This can lead to confusion and misuse of data.
As smaller and mid sized companies grow, they may encounter scalability issues with their data processes and infrastructure. Legacy systems may struggle to handle increasing data volumes and complexity. Additionally, the lack of proper documentation for data sources, definitions, and transformations can make it difficult for employees to understand and trust the data they are working with. Slow data retrieval and processing times can impede operational efficiency. Teams may have to wait for data they need to make decisions or carry out tasks.Not all employees may have access to the data they need to perform their roles effectively. This can hinder collaboration and slow down decision-making processes.
These are all common growing pains which can be managed and mitigated with effective data governance structures. To address these inefficiencies, companies should devote resources towards a data strategy that includes data integration, data governance, data quality management, and analytics capabilities. Investing in modern data management tools and practices can help streamline data processes, improve data accuracy, and support informed decision-making.
What Should You Include in your Data Governance Framework?
1. Data Governance Charter and Policies: a clear and concise data governance charter that defines the purpose, goals, and scope of data governance within the organization. Data governance policies, meanwhile, should outline the principles, standards, and rules governing data management, security, and privacy.
2. Data Governance Committee: Establish a data governance committee composed of stakeholders from different departments, including IT, business, legal, compliance, and data management roles. This committee tends to be larger and more complex in larger organizations; however, even small companies should involve representatives from legal, IT, and a business team in order to account for all of the different considerations of data governance at the company.
3. Data Stewards: appoint data stewards responsible for specific data domains or assets. Data stewards are accountable for data quality, integrity, and compliance within their areas. This helps provide accountability for the different areas encompassed by the overall data governance framework.
4. Data Inventory and Catalog: maintain a comprehensive inventory of data assets and create a data catalog that provides metadata and documentation for each data asset. This can be fairly resource intensive, especially at data heavy large organizations. If this is too unwieldy to tackle, this is a sign that the data governance framework is not doing its job, and there may be areas which can be further simplified or streamlined. In addition to maintaining a catalog, define data classification levels to categorize data based on its sensitivity and criticality to the organization. Ideally, part of this initiative will also be developing guidelines to track data lineage and trace data. This helps the company understand how data flows through the organization, and helps trace data, which is particularly useful when there are conflicting data points.
5. Data Quality and Standards: develop data quality standards and metrics to assess and monitor data quality, consistency, and accuracy. This can be particularly helpful for larger organizations with multiple teams highly involved with data processing; for smaller organizations, this is optional.
6. Data Security and Privacy: align data governance with relevant data protection regulations and privacy laws (e.g., GDPR, HIPAA) and ensure compliance with these laws. Implement procedures for data subject rights and consent management, and establish access control policies and protocols to ensure that data is only accessed and modified by authorized individuals. Implement encryption and other security measures as necessary. This is crucial for organizations of all types and sizes.
7. Data Lifecycle and Change Management: define data retention and archival policies, including data deletion and purging procedures. This is a sometimes forgotten best practice which can lead to cost savings from data storage. Processes should also be established for making changes to data structures, definitions, and data governance policies.
8. Training, Tools, and Processes for Data Governance: as we can see, data governance can be a large scale undertaking. As a result, it should include training programs and communication strategies to educate employees about data governance principles and best practices, as well as technology solutions to automate data governance processes, data lineage tracking, and metadata management. There is a need for internal processes within the data governance umbrella for audits, monitoring of governance practices, and provision of checks and balances on data quality and legal compliance. The data governance committee should define its own KPIs and metrics to measure its effectiveness. This is where a data governance maturity model can also be helpful in periodically assessing effectiveness and setting goals for improvement.
Why Palette?
Automate calculations
Automate sales commission payments. Create commission rules, define a payout schedule and access monthly statements for each sales rep.
Motivate and coach with real time dashboards
Motivate sales professionals with real-time visibility into commissions. Coach your team, align everybody with company goals and drive long term sales performance.
Compare, simulate and design commission plans
Roll out new commission plans with ease. Compare plans and simulate new rules with a single click.
No more errors
Palette keeps history logs and tracks every calculation detail, helping you to reduce sales commission errors.
Reconcile revenue with CRM data
With Palette you can reconcile invoices and payments with CRM data and pay commissions to your reps only when the money is in the bank.
Create challenges with one click
Incentivize your teams on short term goals. Create a challenge targeting any KPI you want to uplift, drive better results and boost your company’s culture.
Loved by teams and companies you know


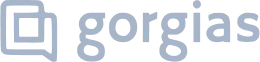







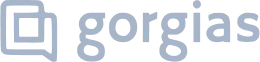






Ready to try
Palette?
